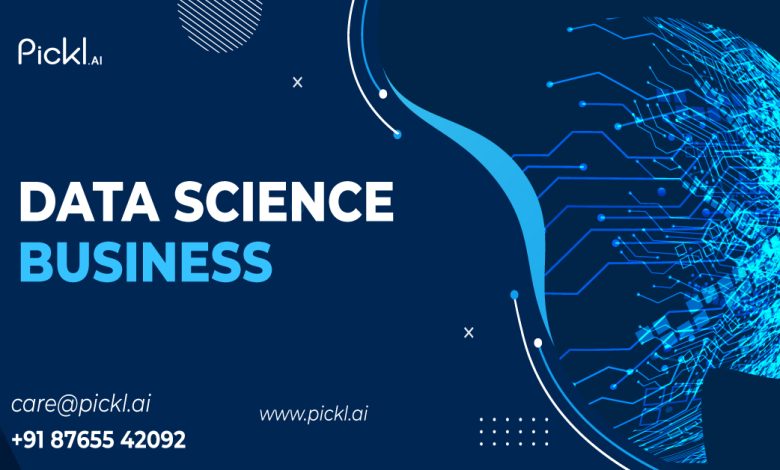
Everyone uses data and in today’s world, it is a resource and fuel that is necessary for industries. It processes unstructured data into a valuable output and uses it to derive insights for the industry’s improved operation. The nexus for data is finance. As early adopters and innovators of data analytics, financial institutions were among them.
Algorithmic trading, customer management, fraud detection, and risk analytics are just a few examples of the many fields where data science is frequently applied. We’ll discuss every detail, offer you some excellent Data Science applications in the finance industry, and then move on.
Deployment of Data Science in the Finance Sector
Risk Assessment
Risk analytics is one of the essential applications of business intelligence and data science in finance. For example, a corporation may make strategic decisions and improve its credibility and security with the help of risk analytics and management.
Data is the foundation of risk management because it calculates loss frequency and multiplies it by the severity of harm. Risk management requires proficiency in arithmetic, statistics, and problem-solving as a cross-disciplinary discipline. Spreadsheets could always handle typical organized data, but more sophisticated data is unstructured. Institutions have several opportunities thanks to this kind of extensive data.
A business might be exposed to many different kinds of hazards. Competitors, credit, the market, etc., are the sources of these hazards. Therefore, identifying, monitoring, and prioritizing risks are critical processes in risk management.
Data like consumer information and financial transactions are readily available in large quantities. The institutions use this data to train themselves to improve risk score algorithms and save expenses. Verifying a customer’s creditworthiness is an essential component of risk management. Businesses determine a customer’s trustworthiness by employing data scientists who examine consumer transactions using machine learning algorithms.
Real-Time Analytics
Batch processing was used in classical analytics to process data. In other words, processing material that was just historical and not real-time. This hampered the need for real-time data in several businesses to acquire insights into the current situation.
But now, it is feasible to get the data without the slightest delay, thanks to technological developments and the creation of dynamic data pipelines. As a result, institutions can track transactions, credit ratings, and other financial parameters with zero latency thanks to this application of data science in finance.
Consumer Analysis
Financial companies focus heavily on customer customization. Data scientists can get information from customer behaviour and make wise business actions using real-time analytics.
Financial organizations like insurance firms use consumer analytics to optimize losses by measuring client lifetime value, increasing cross-sales, and reducing below-zero consumers.
Data Management for Clients
Institutions of finance require data. In actuality, big data has entirely transformed the way financial institutions operate. Social media and many transactions contribute to the quantity and variety of data. The information available is of two types:
- Organized data
- Unorganized data
Although organized data is more straightforward, unorganized information is more problematic. Many NoSQL solutions may come in handy to manage this complex data, and MapReduce can analyze it.
Big Data’s most crucial component is business intelligence. Firms and Businesses use Machine learning to collect business knowledge and produce insights about their consumers. Artificial intelligence (AI) uses various technologies to extract useful information from data, including text analytics, data mining, and natural language processing. For example, a detailed study of the consumer data is used by machine learning algorithms to assess financial patterns and alterations in market prices.
Offering Customized Services
Customers should receive individualized services from financial institutions. Financial institutions use various methods to examine consumer data and produce knowledge about their engagements. These organizations also utilize voice recognition and technologies dependent on natural language processing to improve user interaction.
Financial organizations can draw meaningful insights about customers’ demands from consumer data, improving profits. The institutes might then enhance their tactics and offer their clients improved services.
Detecting Fraud
Financial organizations have a lot of anxiety about fraud. As the volume of transactions has expanded, so have the fraud risks. But with the development of big data and analytical technologies, financial institutions can now keep track of scams.
One of the crimes that are attempted the most frequently in financial organizations is credit card fraud. This kind of fraud comes into light because of advancements in algorithms that boosted the accuracy of anomaly detection. Additionally, these detections notify businesses of irregular financial purchases, forcing them to shut the account to reduce losses.
In trade data, various machine learning technologies can spot odd trends and inform financial institutions to look into them further. For example, banks must cope with other insurance-related scams. In addition, organizations can group and separate data trends that appear to be highly questionable using various clustering methods.
Algorithmic Trading
Financial firms’ most crucial component is algorithmic trading. In algorithmic trading, intricate mathematical formulae and rapid calculations aid financial organizations in developing new trading methods.
Data science has grown to be the most crucial aspect of algorithmic trading due to the significant influence that big data has made. Large data channels comprise the data used in algorithmic trading, using a model to quantify and characterize the underlying data streams. The analytical engine aims to anticipate the future market by learning more in-depth information about the massive datasets.
Credit Allocation
A digital footprint is a huge dataset left behind by every individual who visits or registers on a website. However, it has tonnes of essential data. Machine learning algorithms may parse digital footprints to reveal previously hidden correlations between new parameters and consumer behaviour, assisted by massive data and powerful computing. These findings may impact credit allocation, which is more accurate at estimating a customer’s likelihood of repaying a loan than conventional credit scoring methods.
How to Become a Data Scientist in the Finance Sector?
The job of a data scientist may need a variety of skills in finance. As a result, rather than concentrating on a particular ability, you should try to develop a broad set of functional skills to become a data scientist. Following your hobbies, you may then acquire your talents even further.
You may learn the methods and best practices for integrating data science models into financial processes by specializing in the finance area of data science. Predictive analytics applications in finance are part of the Data Science Course syllabus, along with essential financial strategies and workflow optimization. In addition, online and offline data science courses are readily accessible.
Conclusion
Data science has grown significantly in importance in finance, primarily employed for improved risk management and analysis. More robust analysis results in better judgments and more intelligent choices result in more profits for financial organizations.
Companies also use business intelligence technologies to examine data patterns. The application of data science has increased the precision of anomaly and fraud identification. Due to this, losses have been kept to a minimum, and the financial institution’s image has been preserved while reducing dangers and scams. There are various Data Science Courses available to develop and strengthen yourself for the future if you’re interested in learning data science to stay ahead of rapid technological breakthroughs.
Reason for data analytics training in 2022